STE Highlights, October 2021
Awards and Recognition
Accelerator Operations and Technology
An adaptive approach to machine learning for compact particle accelerators
Computer, Computational and Statistical Sciences
Special issue paper reviews CoPA particle application advancements
Earth and Environmental Sciences
EES-led Large University Project geology study connects damage to permeability, trains students
Awards and Recognition
Researcher joins UCSD in first Laboratory joint appointment with a UC campus

Rodman Linn
A joint appointment brings Los Alamos National Laboratory Scientist Rodman Linn to a five-year position with the University of California San Diego at the Halıcıoğlu Data Science Institute (HDSI). Linn’s expertise lies in solving and modeling problems involving complex thermal, mechanical and fluid dynamics systems, including wildfire behavior. This is the first joint appointment program between the Laboratory and a UC campus.
The University of California is a partner in DOE’s managing and operating contract for Los Alamos. Although interest in joint appointments between the UC-affiliated national laboratories and the UC campuses goes back decades, the establishment of the Southern Hub in 2020 helped put the pieces into place. The hub is a consortium of the five southern UC campuses and the three UC-affiliated national laboratories to create a collaborative network that will enhance research partnerships and education and support a workforce pipeline to the three laboratories.
Linn joins the UCSD as a professor in the Halicioğlu Data Science Institute and as associate director of fire science for the WIFIRE program, a cyberinfrastructure platform at San Diego Supercomputer Center (SDSC) that integrates data systems to help monitor, predict and mitigate wildfires. Historically, WIFIRE has been focused on fire response and data integration to support decision-makers with real-time data models, but now they are contemplating how to use novel data models to support a more proactive approach to fire management, including prescribed burns.
Dr. Ilkay Altintas, chief data science officer at the San Diego Supercomputer Center and a founding fellow of the Halicioğlu Data Science Institute at UC San Diego, is joining LANL as a joint faculty appointee as a part of the Information Science & Technology Institute in NSEC in collaboration. Altintas will be partnering with Linn, and other researchers from EES, NSEC, A and CCS divisions to increase the use of novel data integration, artificial intelligence and simulation modeling to improve the science basis for wildland fires.
Leveraging the skills and talents of HDSI students and faculty also provides a novel approach to using data science in addressing wildfires. HDSI was designed to bring together UC San Diego faculty and researchers, industry partners and other professionals, to identify problems and offer real-world solutions to address critical challenges.
Prescribed burns or controlled burns are a critical tool used to manage wildfires. Prescribed burns are ignited to reduce hazardous fuel loads near developed areas, manage landscapes and restore natural woodlands. In addition to clearing away fuel, prescribed fires can reduce invasive species and increase biodiversity.
In many forested areas today, there is an overabundance of vegetation and dead material build-up, which acts as fuel in wildfires, often increasing the intensity and size. Now the build-up needs to be cleared and ecosystems need to be rebalanced.
To effectively control prescribed burns and optimize their use, forest managers needs to understand how environmental conditions and ignition techniques work together in a fire and how to interpret and control the variables. This is where Linn’s work and the work of Altintas will come together through data integration and analysis and computer modeling.
The joint appointments between UCSD HDSI and Los Alamos include the option to renew. More information can be found here.
Dean, Morales, Li recognized for high energy nuclear physics contributions at RHIC
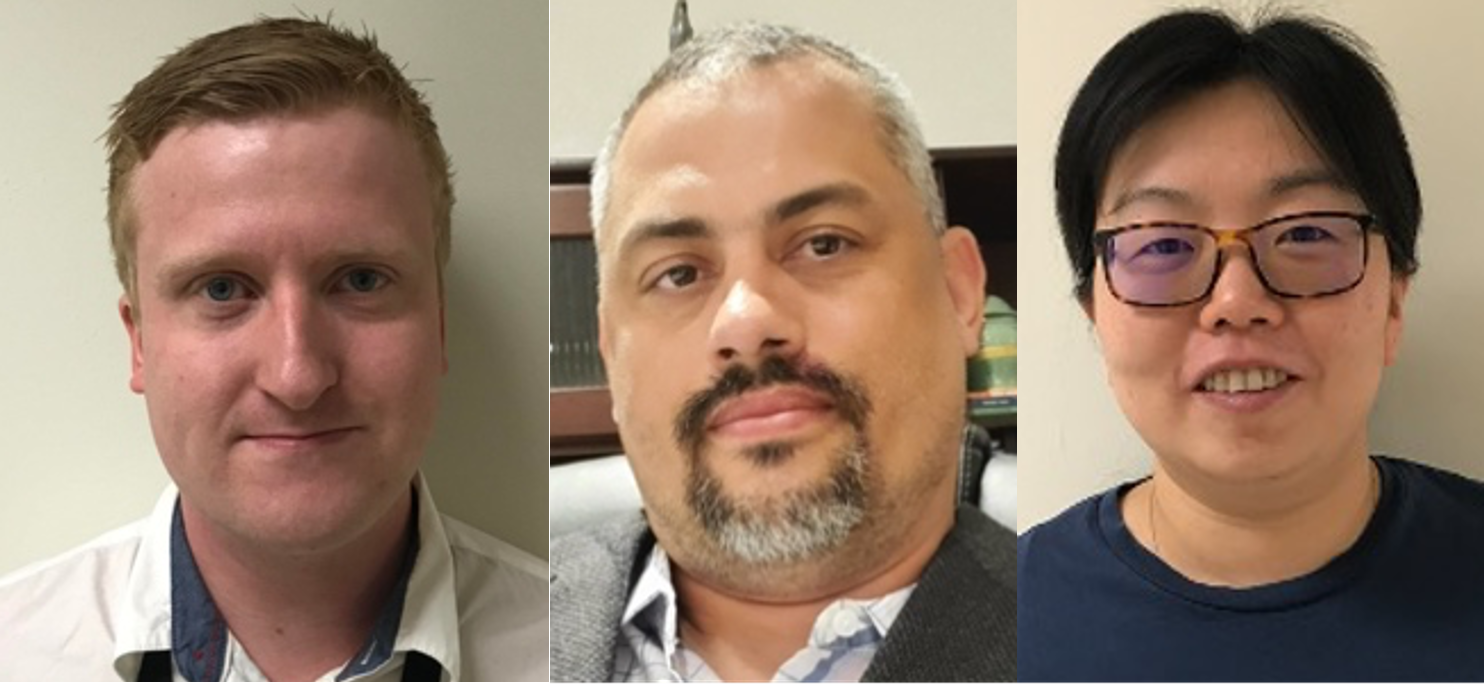
Award winners (left to right) Cameron Dean, Yasser Morales, and Xuan Li.
For their outstanding high energy nuclear physics research and contributions to related programs at the Relativistic Heavy Ion Collider (RHIC), Cameron Dean, Yasser Morales, and Xuan Li received 2021 Merit Awards from the RHIC/AGS Users’ Executive Committee.
Dean was cited “for his essential contributions to the heavy flavor program in sPHENIX through simulations as well as software and detector development.” Morales was cited “for his outstanding contributions to the sPHENIX Monolithic-Active-Pixel-Sensor based Vertex Detector project.” Li was recognized “for her exceptional contributions to the understanding of hadronic structure and nuclear matter with PHENIX, and her leadership in developing a heavy quark program at the future Electron-Ion Collider.” All are members of the High Energy Nuclear Physics Team in Nuclear and Particle Physics and Applications (P-3).
Under construction at RHIC at Brookhaven National Laboratory, sPHENIX is a detector designed to study quark-gluon plasma using jet and heavy-flavor observables. PHENIX (for Pioneering High Energy Nuclear Interaction Experiment) was designed to study high energy collisions of heavy ions and protons. Analysis of the data generated is underway.
Postdoctoral researcher Dean joined the Lab in 2019. His work focuses on vertex development for sPHENIX, heavy flavor analysis for sPHENIX and LHCb—the Large Hadron Collider beauty experiment, and simulations for the Electron-Ion Collider. He earned his PhD in experimental particle physics from the University of Glasgow in 2019.
Postdoctoral researcher Morales, who joined Los Alamos in 2018, assists with sensor readout and data acquisition and hardware research and development for the sPHENIX experiment. He earned his PhD in 2015 at the University of Turin working with the A Large Ion Collider Experiment (ALICE) at CERN.
Staff scientist Li has applied advanced silicon detector technologies for fast, hard x-ray imaging at synchrotrons since 2018 and has been the co-principal investigator of the LANL Electron-Ion Collider Laboratory Directed Research and Development project since 2019. She received her PhD in high energy and nuclear physics in 2012 from Shandong University and completed her thesis research at Brookhaven National Laboratory.
Accelerator Operations and Technology
An adaptive approach to machine learning for compact particle accelerators
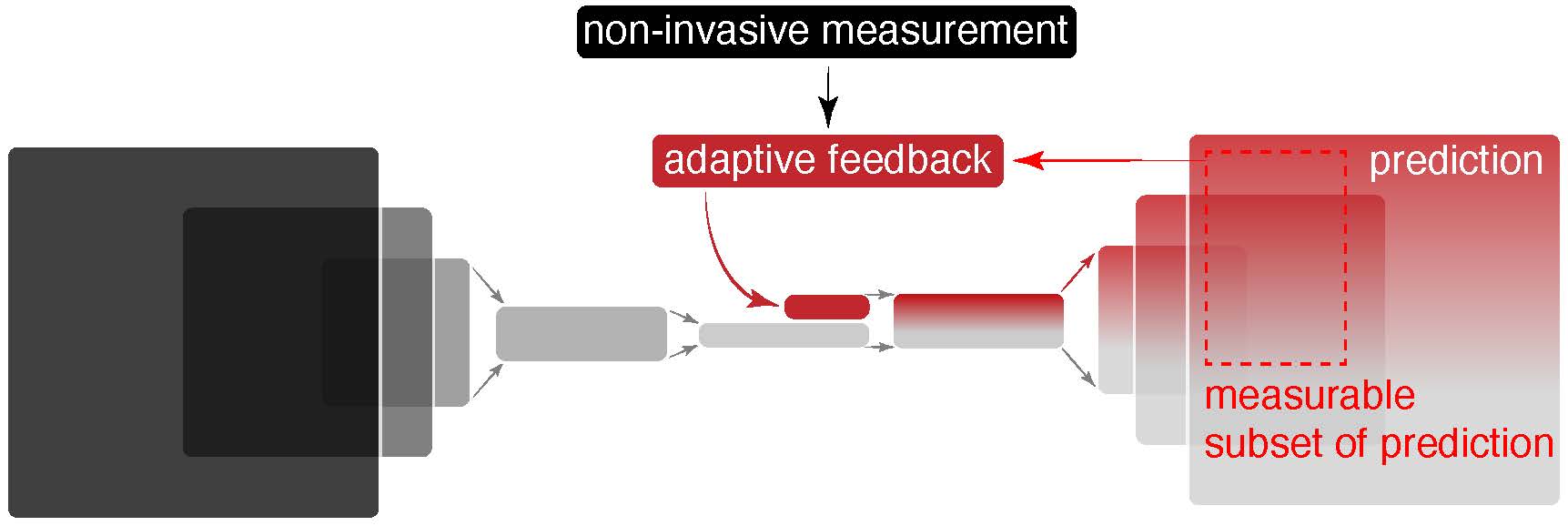
An adaptive approach to machine learning is shown with an encoder-decoder convolutional neural network (CNN) architecture. Extremely high dimensional inputs are mapped down to an incredibly low dimensional latent space representation. A subset of the CNN’s generated prediction is then compared to an online non-invasive diagnostic to guide adaptive feedback in the low-dimensional latent space.
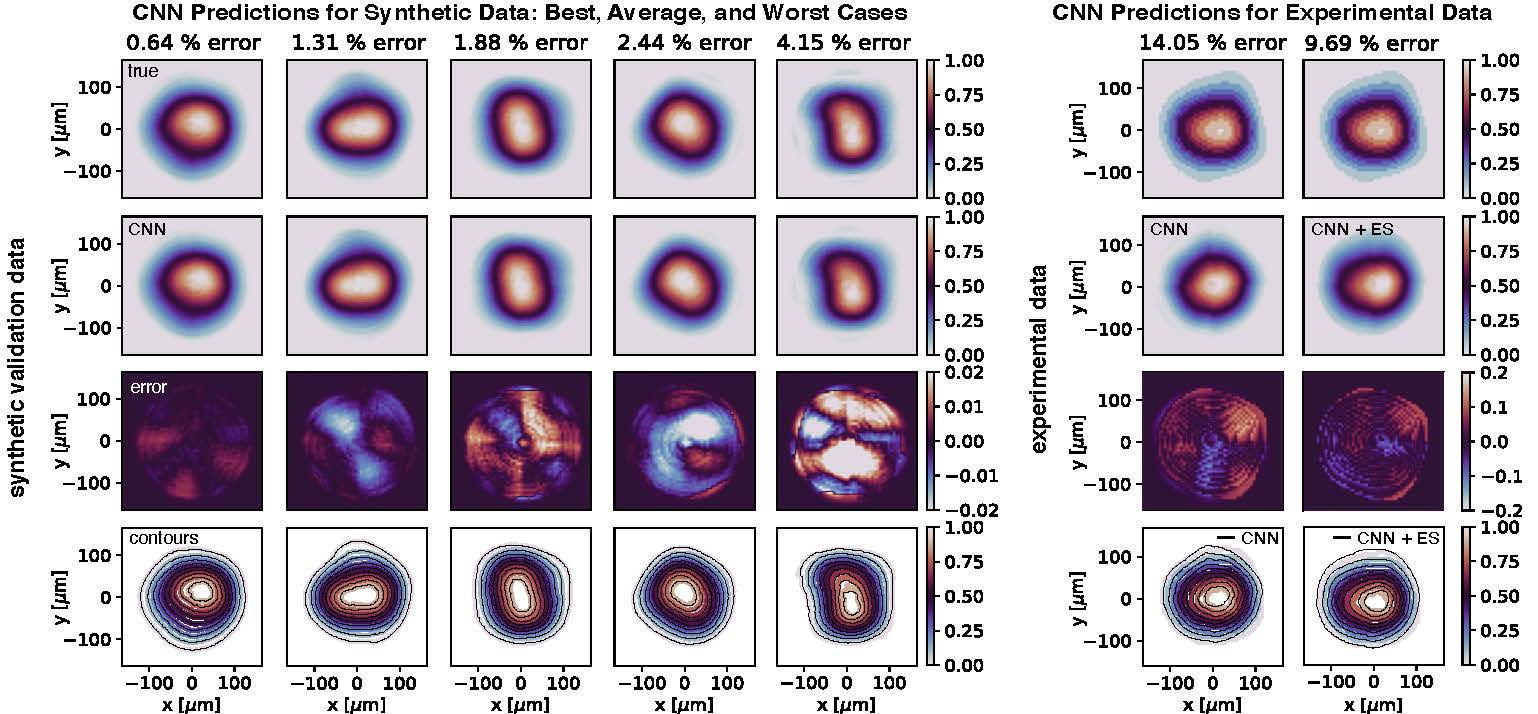
The CNN’s predictive power is shown with close matches of synthetic input beam distributions based only on their output measurements. Right: The CNN is shown to accurately reproduce the experimentally measured input beam distribution based on the measurement of the associated output beam.
Led by Alexander Scheinker, researcher in the Applied Electrodynamics group at Accelerator Operations and Technology, researchers have developed an adaptive approach to machine learning for compact particle accelerators. The main goal of the Los Alamos work is to develop adaptive machine learning algorithms for non-invasive diagnostics and optimal feedback control of time-varying systems with un-modeled disturbances. The research, conducted on the input and output beam distributions of the HiRES ultra-fast electron diffraction (UED) beam line at Lawrence Berkeley National Laboratory, was recently described in Nature’s Scientific Reports.
Compact UED accelerators pose a challenge for machine learning because they are time varying systems in which accelerator components themselves can produce noise – temperatures, small vibrations, magnetization changes, slight variations in energy – that potentially impact the results of experiments which rely on incredibly precise timing (10-100 femtoseconds). Such small variations usually average out or are unimportant for experiments in larger accelerators. An adaptive machine learning approach allows researchers to understand what is happening in the beam and develop advanced ways to control and stabilize the inputs, resulting in more precise measurements for a given experiment.
Machine learning tools offer predictive capabilities using data derived from the relationships between systemic inputs and outputs. Typically, if a system changes the machine learning models require re-training, but re-training methods are impractical for particle accelerators for which detailed beam measurements are slow and interrupt operations. Furthermore, even if a perfect machine learning or physics-based model was available, the beam entering a particle accelerator is itself uncertain and time varying and so the initial conditions to be used for the model are typically unknown.
The approach tested by Scheinker and collaborators uses adaptive feedback in the architecture of deep convolutional neural networks (CNN) to create an inverse model to predict input beam distributions based on downstream measurements which can be carried out more easily without interrupting accelerator operations. The method employs an extremum seeking (ES) model independent feedback algorithm based on a measurement of error to adaptively tune the CNN’s predictions as the system varies with time without relying on re-training.
The approach relies on taking measurements downstream of the input accelerator beam, so as to not interrupt operations. The inverse model maps back to predict what the input beam is at any instant of time. The adaptive CNN is coupled with an online physics-based model which uses the predicted initial beam distribution as input to simulate the detailed beam evolution throughout the entire compact accelerator. Because the system is time varying, the physics-based model is itself also adaptively tuned based on the error between its predictions and output beam measurements.
This hybrid approach of coupling an adaptive CNN together with an adaptive physics model allows the system to simultaneously track changes in both the unknown input beam distribution as well as in time-varying accelerator components, such as magnet currents, by iterative feedback that makes adjustments in real time for time-varying characteristics of the compact accelerator.
The CCN+ES approach offers advantages for non-invasive beam diagnostics. The feedback can be used for real-time beam tuning, allowing for rapid movement between experiments, or applying controls to beam lines. The approaches for compact accelerators may also be expanded upon for application to larger accelerators. The problem of time-varying characteristics and tuning is relevant to all large complex systems and in particular to all complex accelerators including LANSCE, DARHT, APS, the LCLS-I/II, and to projects such as the Dynamic Mesoscale Materials Science Capability, with short pulses of X-rays.
Funding and Mission
This work is supported by the U.S. Department of Energy (DOE), Office of Science, Office of High Energy Physics. The work supports the Global Security mission area and the Nuclear and Particles Futures capability pillar.
Reference
"An adaptive approach to machine learning for compact particle accelerators," Scientific Reports, 11, 19187 (2021). DOI: https://doi.org/10.1038/s41598-021-98785-0. Authors: Alexander Scheinker (AOT-AE), Frederick Cropp, Sergio Paiagua, and Daniele Filippetto.
Technical Contact: Alexander Scheinker
Chemistry
Digestion and Trace Metal Analysis of Uranium Nitride
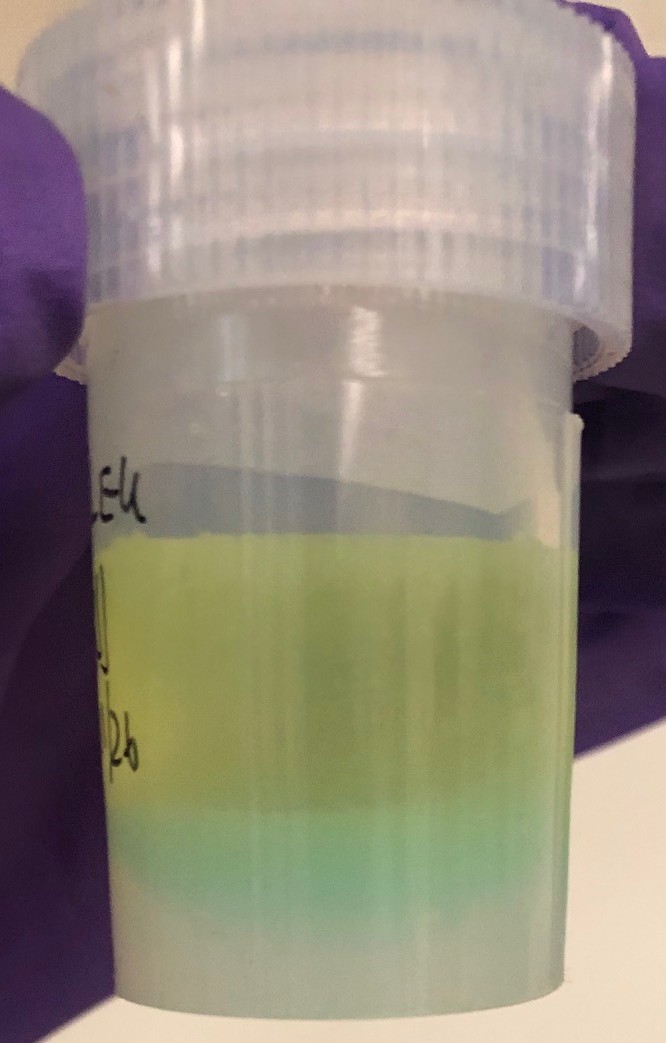
A partially digested uranium nitride sample.
Scientists in the Chemical Diagnostics and Engineering group (C-CDE) have been developing a novel digestion method and characterization of uranium nitride fuel for use in advanced reactors, microreactors, and nuclear thermal propulsion. Led by principal investigator Tim Coons, in the Materials Science in Radiation and Dynamic Extremes group (MST-8), the work supports a larger project to develop reliable energy sources for a variety of purposes including a manned mission to Mars.
Characterization of minor impurities for novel nuclear fuels is necessary for the safety and reliability of irradiation testing, which will be used to accurately predict fuel performance in-pile. Much of the research to date on fast reactor fuels has targeted synthesis of uranium nitride. The CDE work developed and optimized destructive assay procedures for fresh fuel characterization of trace impurities and isotopic analysis for chemical quality control. The researchers completely digested uranium nitride. Using column separation, 47 analytes were quantified as potential impurities and percent recoveries were calculated via inductively coupled plasma mass spectrometry.
The first digestion matrix tested comprised of 8M HNO3-0.2M HF. This matrix has been known to readily dissolve uranium oxide and is used as the loading solution for the column separation. After heating for approximately 12 hours, it was observed that the sample had not fully digested. The sample had separated into two layers; a yellow solution indicative of uranyl nitrate and a turquoise solid. The analysis of the solid precipitate confirmed the main phase was uranium tetrafluoride (UF4). When performing a mass balance of the fluorine content in the reaction, the total amount of UF4 precipitated equals the amount of fluorine moles from the HF added in the solution. This is a surprising novel synthesis for UF4. Normally UF4 needs anhydrous environments to preferentially form. Alas, the formation of UF4 is an undesirable result for a chemical quality control destructive assay method, as all of the sample needs to be digested. A second acid digestion was performed by sequentially adding the acids, which resulted in one single phase. It is important that all of the nitride is converted to uranyl nitrate before the addition of HF.
In order to validate the digestion and column method, the percent recovery of trace elements with known quantities of 47 elements was performed. Overall, the separation method successfully recovered 90% or greater of all the elements tested, except for magnesium (85%). The digestion, separation, and quantification of trace metals were optimized and demonstrated for uranium nitride as a proposed destructive assay method for chemical quality control of fresh fuel.
Funding and Mission
This work was funding through NASA-Space Nuclear Propulsion. The program’s mission is to create a sustainable presence on the moon and send astronauts to Mars. It supports the Energy Security mission area and the Materials for the Future capability pillar.
Technical Contact: Keri Campbell
Computer, Computational and Statistical Sciences
Special issue paper reviews CoPA particle application advancements
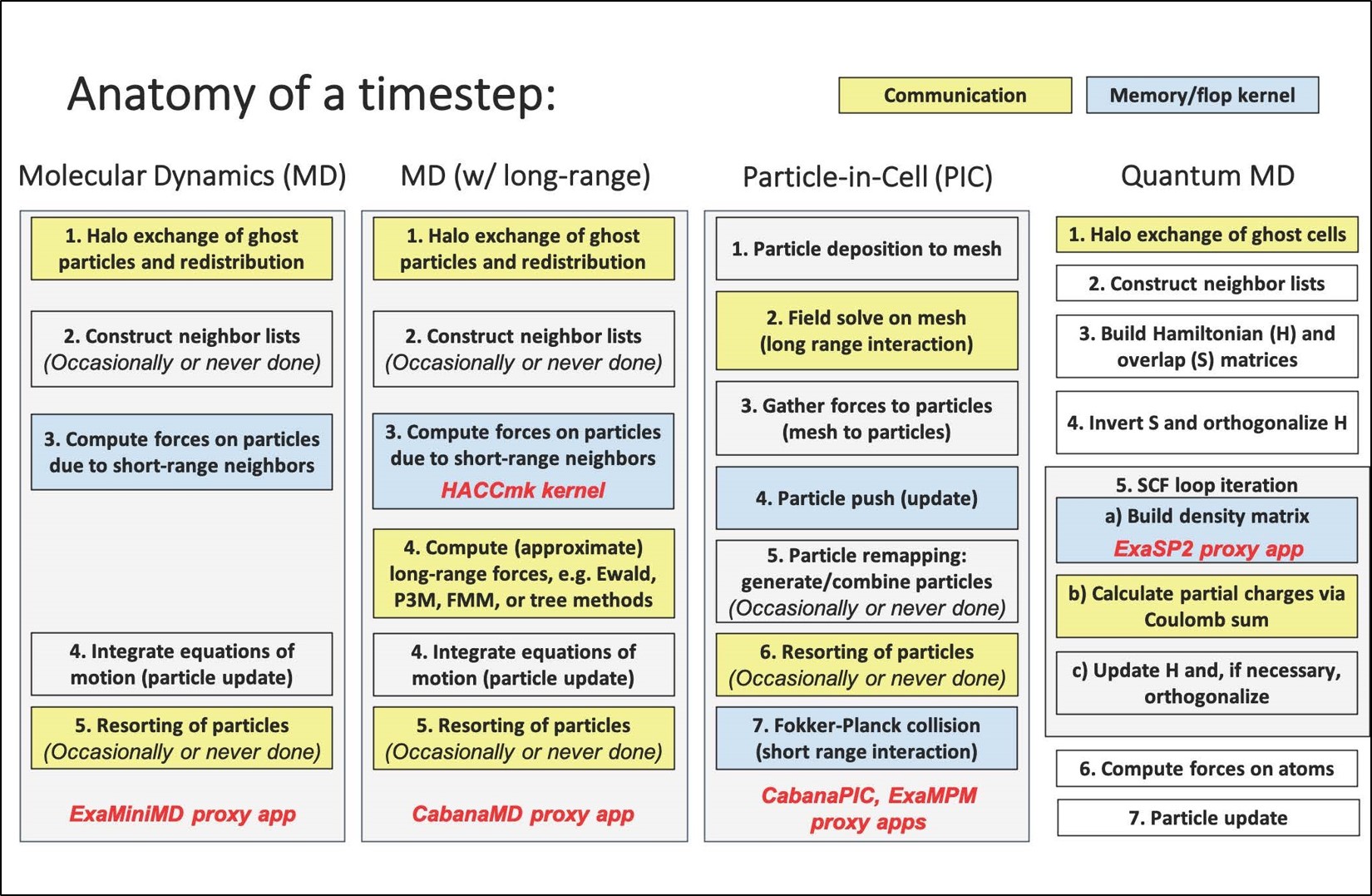
Anatomy of a time step is shown for each of the particle application submotifs addressed by the Exascale Computing Project’s Co-design Center for Particle Applications. Communication-intensive steps and compute/memory-intensive steps are shown in yellow and blue, respectively.
The Exascale Computing Project’s (ECP’s) Co-design Center for Particle Applications (CoPA) aims to prepare particle applications for exascale computing. A recent article in a special issue paper of the International Journal of High Performance Computing Applications by CoPA researchers, with Laboratory researcher Susan M. Mniszewski the lead author, among other Lab contributors and researchers from other laboratories and universities, reviews particle application developments at the Center.
CoPA provides proxy applications (apps) and libraries that enable exascale readiness. CoPA’s research focuses on submotifs that address (1) short-range particle–particle interactions, which often dominate molecular dynamics (MD) and smoothed particle hydrodynamics methods; (2) long-range particle–particle interactions, used in electrostatic MD and gravitational N-body methods; (3) particle-in-cell methods; and (4) linear-scaling electronic structure and quantum molecular dynamics (QMD) algorithms.
Proxy apps such as CoPA-developed ExaMiniMD, CabanaMD, CabanaPIC, and ExaSP2 are used to evaluate the viability of incorporating various algorithms, data structures, and architecture-specific optimizations and associated trade-offs. CoPA’s two main libraries—Cabana Particle Toolkit and PROGRESS/BML QMD Libraries—optimize data structure, layout, and movement to deliver performance portability, flexibility, and scalability across architectures with and without GPU acceleration. The Cabana Particle Toolkit applies to short-ranged, long-ranged, and particle-grid interactions, while PROGRESS/BML QMD Libraries provide methods and solvers for quantum mechanical interactions. The libraries are used by ECP and non-ECP application partners. CoPA includes members from the following ECP partner applications: WDMapp/XGC (Princeton Plasma Physics Laboratory), EXAALT/LAMMPS/LATTE (Sandia National Laboratory, LANL), ExaSky/HACC (Argonne National Laboratory), and ExaAM/PicassoMPM (Oak Ridge National Laboratory).
CoPA projects have shown that proxy apps are beneficial for rapid prototyping of different ideas and performance speedup and that co-design teams of domain scientists, computational scientists, and expert programmers in hardware-specific languages and programming models is an effective work mode for advancing particle applications. Specific project impacts include the transitioning of XGC from FORTRAN to C++ using the Cabana Particle Toolkit and Kokkos and the development of a hybrid quantum mechanical/molecular mechanical QM/MM protein simulation capability, which has proven useful in biomedical research including studies of SARS-CoV-2 proteins.
CoPA’s ongoing work on particle application readiness for exascale computing includes using Cabana/Kokkos in XGC for GPU off-loading of the ion particle operation in the ITER tokamak for performance-portable simulations on exascale platforms; improving additive manufacturing simulations with the PicassoMPM code; and developing HACC-based proxy apps towards a potential full N-body cosmological simulation code.
Mission and Funding
This work was supported by the Department of Energy, and performed as part of the CoPA, supported by the Exascale Computing Project (17-SC-20-SC), a collaborative effort of the DOE, Office of Science and the NNSA. The work supports the Global Security mission area and the Information Science and Technology capability pillar.
Reference
“Enabling particle applications for exascale computing platforms,” The International Journal of High Performance Computing Applications, 0, 0, 1-26 (2021); DOI: 10.1177/10943420211022829. Authors: Susan M. Mniszewski, Michael E. Wall (CCS-3); Adetokunbo A. Adedoyin, Christoph Junghans, Jamaludin Mohd-Yusof, Robert F. Bird (CCS-7); Guangye Chen (T-5); Christian F. A. Negre (T-1); Shane Fogerty (XCP-2); Salman Habib, Adrian Pope (ANL); James Belak, Daniel Osei-Kuffor, Lee Ricketson (LLNL); Jean-Luc Fattebert, Stuart R. Slattery, Damien Lebrun-Grandie, Samuel Temple Reeve (ORNL); Choongseok Chang, Stephane Either, Amil Y. Sharma (PPPL); Steven J. Plimpton, Stan G. Moore (SNL); Aaron Scheinberg (Jubilee Development).
Technical Contact: Susan Mniszewski
Earth and Environmental Sciences
EES-led Large University Project geology study connects damage to permeability, trains students

The Large University Program project united theoretical, modeling and experimental capabilities.
As part of the Large University Project, a three-year collaborative program, “Correlating Damage, Fracture and Permeability in Rocks Subjected to High Strain Rate Loading” has improved understanding of geological processes while providing University of New Mexico students opportunities to participate in a Laboratory work environment. The integrated program united the Earth and Environmental Sciences (EES) division’s world-class theoretical and modeling capabilities at Los Alamos with state of-the-art experimental capabilities at UNM.
The experiments were directed at improving the understanding of rock dynamics, damage and fracture processes, and the resulting changes in permeability and fluid flow. In particular, novel, customized experiments and accompanying analyses incorporated the rock’s loading rate dependence, a critical aspect of rock damage and fracture.
EES Scientist Esteban Rougier led the study in collaboration with co-principal investigator Earl Knight and University of New Mexico professors and graduate students. To evaluate the material models, participants used the EES Division-designed computational geomechanics software, the Hybrid Optimization Software Suite (HOSS), as well as the Laboratory’s High Performance Computational platforms. HOSS, an R&D 100-award finalist, is a code based on the combined finite-discrete element methodology and was developed to study fracture and fragmentation around underground explosions placed in a wide variety of rock media. Since its inception in 2004, HOSS has been extended to many other fields of applications, such as grain scale mechanical response of cementitious materials, reproduction of laboratory scale experiments for rocks and metals, simulation of hydraulic fracture processes, modeling of dynamic seismic rupture processes, and the study of hypervelocity impacts on both consolidated and unconsolidated materials. The investigation conducted in this project enhanced LANL’s capabilities to predict damage, fracture and resulting permeability changes in geologic materials. By the end of the project the HOSS code was further verified and validated for these type of applications.
Importantly, the study establishes connections between the damage suffered by the rock samples and the changes in their permeability, which are key for many subsurface-based activities such as oil and gas extraction, CO2 sequestration, geothermal energy production, and underground nuclear detonation detection, just to name a few. Establishing the link between permeability and damage will allow more efficient and realistic design of methods for energy extraction (e.g., hydrofracturing, enhanced geothermal stimulation). Rock often provides isolation between stored or disposed of materials and the environment (e.g., hydrocarbon storage, nuclear waste disposal); better understanding of permeability changes in the rock surrounding these facilities will lead to improved confidence in our ability to design and operate these facilities in a manner that protects the environment.
The project also provided an invaluable conduit for Northern New Mexico students to gain experience working at a DOE National Laboratory and gain training that could lead to a career at the Laboratory, taking on challenging national and energy security problems. Students who progressed through the program are well-prepared to provide key contributions either to LANL or the commercial workforce. Three students who participated in the project have subsequently been hired at the Laboratory. The first student, hired in the fall of 2019, was recently converted in the summer of 2020 as a staff member. The other two students are in the process of being hired as post-masters researchers.
Mission and Funding
This program was supported by the Center for Space and Earth Sciences and the New Mexico Consortium (NMC; Rougier is also an NMC affiliate) and supports the Energy Security mission area and the Complex Natural and Engineered Systems capability pillar.
Technical Contact: Esteban Rougier