STE Highlights, December 2023
Awards and Recognition
Computer, Computational and Statistical Sciences
Researchers develop model to estimate COVID-19 contagiousness across United States
Earth and Environmental Sciences
Review assesses Arctic erosion and channel development prediction needs
Materials Physics and Applications
Novel machine learning potential developed to understand materials deformation
Materials Science and Technology
Novel deposition technique for reusable vessels in nuclear metallurgical foundries
Awards and Recognition
Guo elected to lead APS Topical Group in Plasma Astrophysics
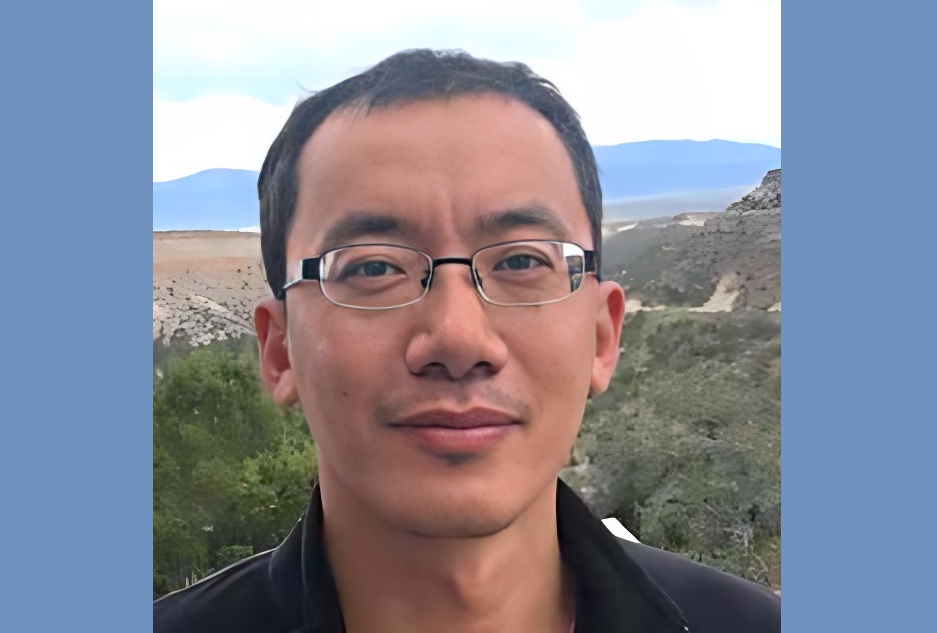
Fan Guo
Fan Guo, physicist in the Nuclear and Particle Physics, Astrophysics and Cosmology (T-2) group at Los Alamos National Laboratory, has been elected to lead the American Physical Society’s Topical Group in Plasma Astrophysics (GPAP). The four-year leadership role starts with the position of vice chair, followed by the positions of chair-elect, chair and past-chair.
The Topical Group on Plasma Astrophysics was formed in 1999 to provide an intellectual home for plasma physicists who have an interest in astrophysical phenomena. Plasma astrophysics represents an interdisciplinary body of knowledge that seeks common ground between plasma physics and astrophysics. The discipline involves the application of fundamental concepts of plasma physics to the solution of outstanding problems in astrophysics.
Guo has also served as an executive committee member of NASA Living with a Star Program Analysis Group since 2018. He is a member of the NASA-funded Solar Energy Flare Release and SHIELD DRIVE Science centers on heliophysics, as well as a team member of NASA’s Interstellar Mapping and Acceleration Probe mission for exploring plasma environments in the inner and outer heliosphere. He has long been interested in promoting space plasma physics and plasma astrophysics among different communities.Bioscience
Machine learning helps improve toxin prediction
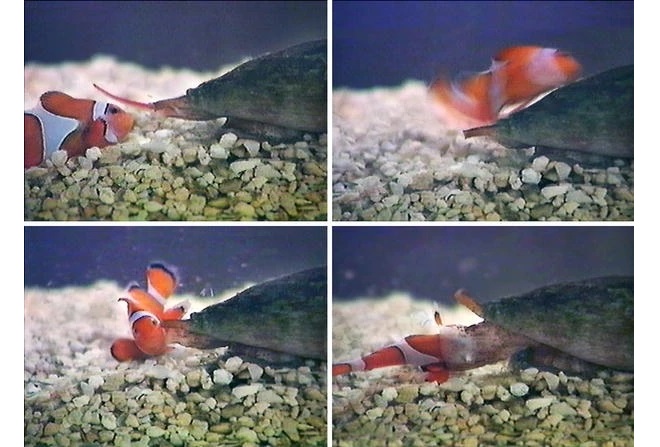
A cone snail releases a conotoxin to paralyze a fish before attacking it. Image from Applied Microbiology and Biotechnology and used via Creative Commons license.
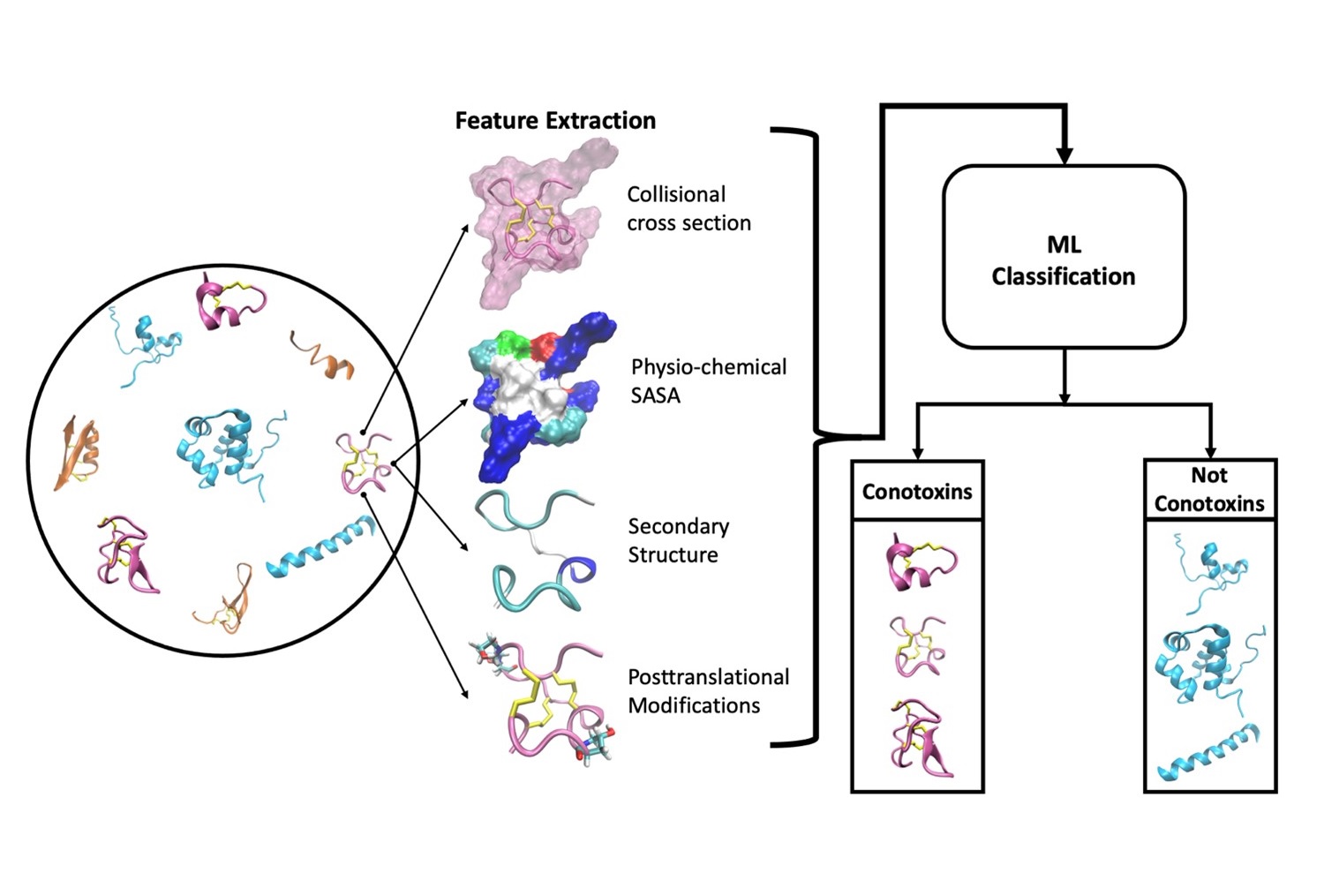
The primary structure of a peptide (a short chain of amino acids) is tied to the amino acid sequence, however, additional modifications such as bonds between sulfur atoms from two cysteine amino acid residues can occur after the peptide is made and can significantly change its structure and function. This graphical abstract shows that by using new additional features — such as collisional cross sections, post-translational modifications — the Los Alamos team improved the machine-learning classification of conotoxins and other kinds of peptides.
Scientists in the Bioscience division, along with collaborators in Theoretical division, published a new paper in the journal Toxins about improvements to predicting conotoxins. In the paper, “Conotoxin Prediction: New Features to Increase Prediction Accuracy,” the authors explain how, by adding new features to a machine learning platform, they improved the platform’s accuracy to predict conotoxins, distinguishing them from any other molecule.
Conotoxins are toxic peptides (short chains of amino acids) found in the venom of carnivorous aquatic mollusks known as cone snails; the snails use these toxins to paralyze their prey. Conotoxins are important to study because they are not only dangerous threats to humans, but also show dual use as potential therapeutics as treatments for cancer, microbial infections, epilepsy, autoimmune diseases, and more.
The challenge for identifying conotoxins in biosecurity and biodefense and for use as therapeutics lies in determining which peptide conformations are more/less toxic and which are not. Typically, the conformational structures of peptides or proteins are dictated by the amino acid sequence that builds the peptide or protein. However, the Los Alamos scientists explain that conotoxins exhibit an unusual amount of additional structural changes caused by disulfide bonds — strong bonds between sulfur atoms — that are often found between two cysteine amino acid residues. Because disulfide bond formation and the resulting conformational changes to the conotoxin are not presented by the amino acid sequence, many existing machine learning algorithms cannot correctly predict conotoxins when relying on primary amino acid sequence as the only features. Furthermore, manual determinations of structure from X-ray crystallography or nuclear magnetic resonance are extremely complicated and time-consuming.
To mitigate this challenge, the Los Alamos scientists trained their machine learning platform with additional experimental information that could help distinguish between conotoxins and non-toxic or other toxic peptides. For instance, the team included physiochemical surface properties, secondary structure characteristics and collisional cross sections of the conotoxins, which can be obtained using the extensive mass spectrometry capability in Bioscience division. The authors demonstrated that by adding these features to the machine-learning platform, the algorithms more accurately predicted conotoxins from other kinds of peptides. The ability to more reliably predict conotoxins will not only help defend against novel biochemical threat agents but also benefit the development of biological prophylactics and therapeutics, detection reagents, and medical countermeasures.
Funding and mission
The work, which supports the Laboratory’s Global Security mission area and its Science of Signatures capability pillar, was funded by Los Alamos’ Laboratory Directed Research and Development program.
Reference
“Conotoxin Prediction: New Features to Increase Prediction Accuracy,” Toxins 15, 11, 641 (2023); DOI: 10.3390/toxins15110641. Authors: Lyman K. Monroe, Duc P. Truong, Jacob C. Miner, Samantha H. Adikari, Zachary J. Sasiene, Paul W. Fenimore, Boian Alexandrov, Robert F. Williams, and Hau B. Nguyen (Los Alamos National Laboratory)
Technical contacts: Lyman Monroe and Hau Nguyen (B-TEK)
Computer, Computational and Statistical Sciences
Researchers develop model to estimate COVID-19 contagiousness across United States
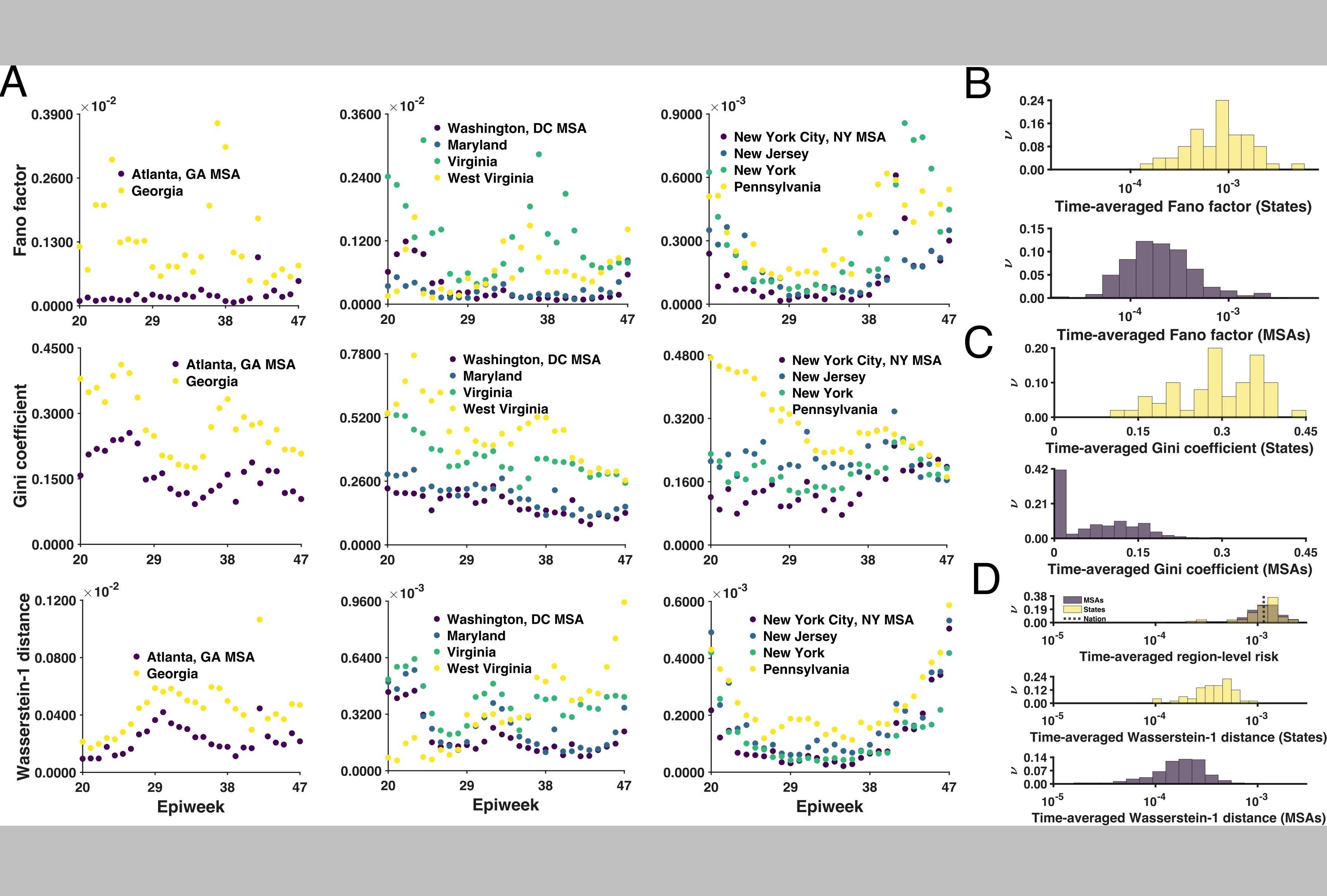
The figure illustrates: (A) variability measures based on the Fano factor, Gini coefficient and Wasserstein-1 distance, respectively, for the metropolitan statistical areas of Atlanta, Georgia, Washington, D.C. and New York; B) time-averaged probability distributions of the Fano factor variability measure; (C) time-averaged probability distributions of the Gini coefficient variability measure; (D) time-averaged probability distributions of infection risks and Wasserstein-1 distance variability measure for states and MSAs. For B, C and D, the y-axis indicates relative frequency.

MAP estimates of the COVID-19 basic reproduction number R0 for the strains of SARS-CoV-2 emerging in 280 MSAs and the corresponding overlapping states in 2020. MSA-specific estimates of R0 are sorted by MSA from largest to smallest values according to the R0 estimates inferred from surveillance data collected between January 21, 2020 and June 21, 2020. MSA-specific estimates of R0 are indicated as filled circles and overlapping state-level R0 estimates (one or more) are indicated as squares. State-level R0 estimates closest to the MSA-level R0 estimate for a given MSA are indicated as filled squares. The whiskers associated with each filled circle indicate the 95% credible interval.
In work described in the journal Epidemics, a Los Alamos research team formulated a mathematical model for COVID-19 transmission, parameterizing the model for consistency with available daily COVID-19 case surveillance data for metropolitan areas across the United States. The model allowed the researchers to obtain estimates of the initial contagiousness of COVID-19 across most urban areas in the United States. As some urban areas are shown to be more susceptible to rapid disease transmission than others, the team’s results may help in managing future outbreaks. The findings may be helpful in identifying which population properties contribute to contagiousness.
The team used their mechanistic compartmental model in concert with COVID-19 surveillance data and Bayesian inference, obtaining region-specific COVID-19 estimates. The research team was able to characterize the uncertainty in each estimate of the basic reproduction number for COVID-19. Across urban areas, those estimates quantify differences in population properties that affect disease contagiousness. The team found that the initial contagiousness of COVID-19 varied over a four-fold range across urban areas of the United States, with metropolitan areas more uniformly impacted by COVID-19 than states.
Reference
“Differential contagiousness of respiratory disease across the United States,” Epidemics, 45, 100718 (2023); DOI: 10.1016/j.epidem.2023.100718. Authors: Abhishek Mallela, Yen Ting Lin and William S. Hlavacek (Los Alamos National Laboratory).
Funding and mission
This work was supported by the National Science Foundation, the National Institute of General Medical Sciences of the National Institutes of Health, and the Laboratory Directed Research and Development program at Los Alamos National Laboratory. The work supports the Global Security mission area and the Complex Natural and Engineered Systems capability pillar.
Technical contacts: William Hlavacek and Abhishek Mallela (T-6 and T-CNLS) and Yen Ting Lin (CCS-3)
Earth and Environmental Sciences
Review assesses Arctic erosion and channel development prediction needs
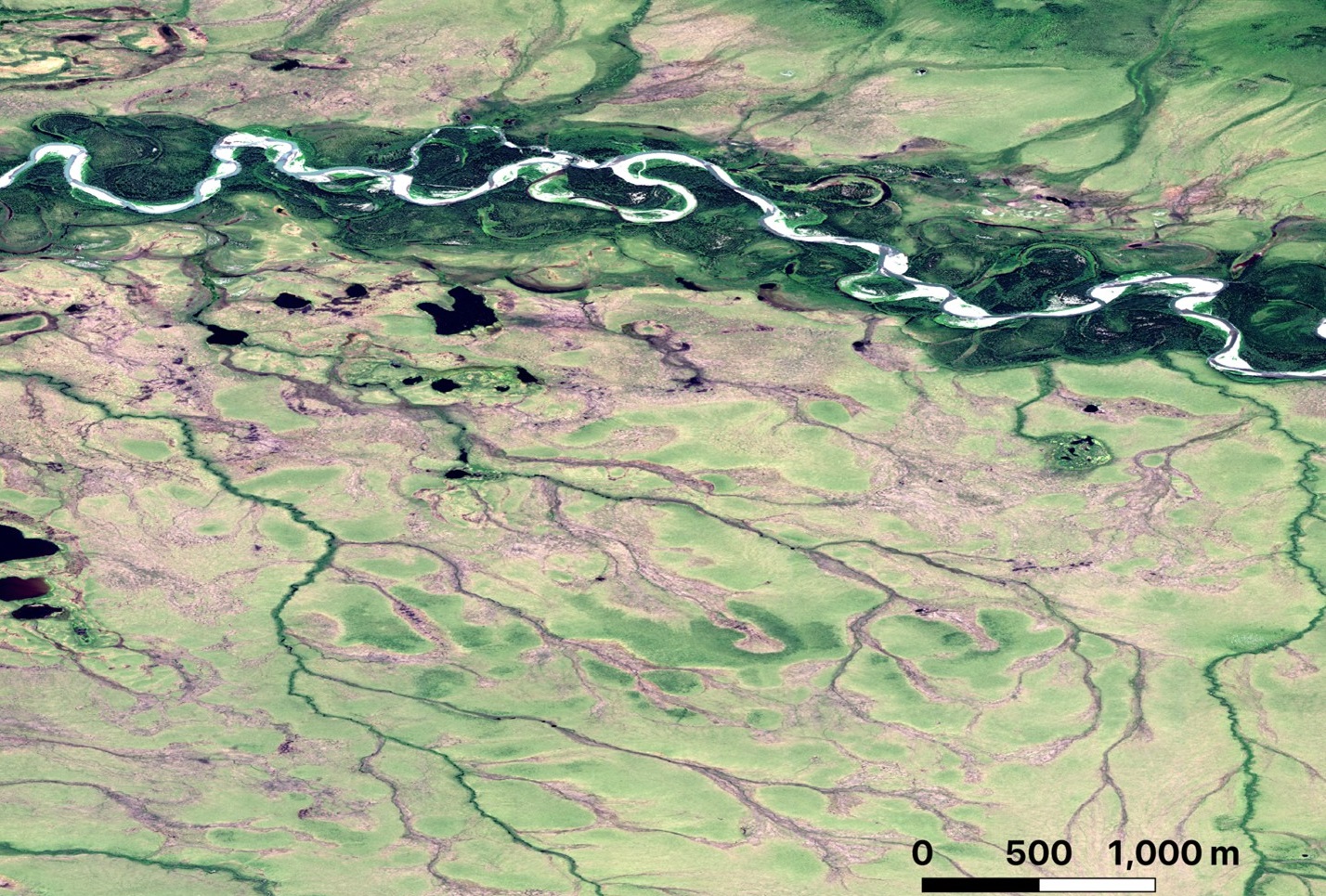
Satellite imagery shows Selawik River drainage networks at various states of development and connectivity. The development of channels follows ice wedge networks, and the ice wedge orientations remain imprinted on even the most developed channels (Maxar copyright 2011).
Despite increasing observations of erosion and channel formation in permafrost watersheds, researchers still lack the predictive tools needed to understand how these changes will alter watersheds in permafrost regions. Such tools can offer critical information on the timing, location and rapidity of permafrost landscape change. Writing in Nature Communications, Joel Rowland (GS-NSD) details factors that affect channel network expansion and hydrological connectivity across Arctic watersheds, describes challenges with current mapping and modeling capabilities, and offers insight into the increasingly detailed, mechanistic and integrated modeling that can enable better predictions.
Channel network expansion is predicted to be caused by thawing permafrost, melting ground ice and changing hydrological regimes. But the widespread evolution of Arctic watersheds due to new channel erosion has not yet been observed. Predicting and quantifying the impact of the thawing Arctic landscapes such as altered fluxes of sediments, carbon and nutrients into waterways is inhibited by the limited applicability of models developed for temperate landscapes that lack key Arctic processes related to permafrost and ground ice.
Studying the causes of rapid erosion in permafrost landscapes will further an understanding of drainage channel formation. Those changes have implications for the routing of carbon and water across Arctic watersheds. Ultimately, that movement results in carbon release from permafrost to the atmosphere, hydrosphere and ocean in ways that warrant better understanding and prediction. As models are developed to accommodate the unique characteristics of permafrost landscapes, the impacts of permafrost disturbance can be incorporated into broader models used to better understand how permafrost thaw and accompanying shifts in Arctic hydrology will alter Arctic landscapes in response to climatic change.
Funding and mission
This work was supported by the Next-Generation Ecosystem Experiments (NGEE Arctic) project, funded by the U.S. DOE Office of Science, Office of Biological and Environmental Research. The work supports the Global Security mission area and the Complex Natural and Engineered Systems capability pillar.
Reference
“Drainage network response to Arctic warming,” Nature Communications, 14 (2023); DOI: 10.1038/s41467-023-40796-8. Author: Joel C. Rowland (Los Alamos National Laboratory).
Technical contact: Joel C. Rowland (GS-NSD)
Algorithm investigates the impact of transported aerosol

Mean percentage change of aerosol number concentration and CCN activation fractions for the three types of long-range transport aerosol events observed at the Eastern North Atlantic by the Atmospheric Radiation Measurement’s Aerosol Observing System in 2017. Image published in Atmospheric Chemistry and Physics and used via Creative Commons license.
As part of an effort to investigate the impact of long-range transported continental aerosol on the regional aerosol regime in the Eastern North Atlantic, a research team including Los Alamos scientists developed an algorithm that helps identify multiday events of such aerosol transport in the region. The results, described in Atmospheric Chemistry and Physics, analyzed the composition of the aerosol plume transport and point to periodic effects on the Eastern North Atlantic by multiday events of long-range continental aerosol transport. The marine boundary layer also was impacted by the multiday events as a significant source of cloud condensation nuclei (CCN). This is important to constrain because CCN are the aerosols that have the potential to form cloud droplets. Clouds alter surface and atmospheric radiation within the Earth system and continue to contribute large uncertainties within global climate models.
The research applied in situ data collected in 2017 at the U.S. Department of Energy Atmospheric Radiation Measurement (ARM) user facility on Graciosa Island called Eastern North Atlantic (ENA). The measurements were a part of the Aerosol and Cloud Experiments in the Eastern North Atlantic (ACE-ENA) campaign and used the Aerosol Observing System (AOS) data. The ENA is a mostly pristine remote marine environment dominated by marine boundary layer clouds, a setting that offered nine multiday events of long-range transported particles in 2017. The algorithm developed by the team is able to integrate three different types of transported continental events: dust and marine particles, polluted mix particles, and biomass burning particles. The analysis showed that biomass burning aerosol events had the largest impact on CCN activation fractions sampled at ENA.
To better understand aerosol perturbation events on CCN regimes, the study provides key observational constraints that can be used to set parameters based on changes in baseline total particle number concentration to determine different aerosol regimes that impact the region. Those constraints can also parametrize the influence of particles sampled from different size modes within the regimes. The results have the potential to inform improvements and validation in climate models.
Funding and mission
The work was supported by the Atmospheric Radiation Measurement (ARM) user facility and funded by the U.S. Department of Energy (DOE), Office of Science, Office of Biological and Environmental Research. The ARM research facility, a DOE Office of Science user facility sponsored by the Office of Biological and Environmental Research, provided data; data and/or imagery from NASA’s Fire Information for Resource Management System (FIRMS) was also used. This work supports the Global Security mission area and the Science of Signatures capability pillar.
Reference
“Long-range transported continental aerosol in the eastern North Atlantic: three multiday event regimes influence cloud condensation nuclei,” Atmospheric Chemistry and Physics, 23, 4221–4246 (2023); DOI: 10.5194/acp-23-4221-2023. Authors: Francesca Gallo, Allison C. Aiken (Los Alamos National Laboratory); Janek Uin, Stephen Springston, Chongai Kuang (Brookhaven National Laboratory); Kevin J. Sanchez, Richard H. Moore (NASA Langley Research Center); Jian Wang (Washington University in St. Louis); Robert Wood (University of Washington), Fan Mei (Pacific Northwest National Laboratory); Connor Flynn (University of Oklahoma); Eduardo B. Azevedo (University of Azores, Portugal).
Technical contact: Allison C. Aiken (EES-14)
Materials Physics and Applications
Novel machine learning potential developed to understand materials deformation

Temperature phase diagram of tin comparing the results from the current potential (lines) to the experimental data available (points).
To design materials for extreme applications, understanding and predicting phase transitions and their influence on material properties under high pressures and temperatures is key. Atomic-scale modeling is a useful tool for assessing these behaviors; however, its accuracy depends on the precision of interatomic potentials — that is, information regarding the interaction of the atoms that make up the material.
In Physical Review Materials, a team of Los Alamos researchers and their university colleagues present a hybrid potential, one that combines the speed of conventional embedded atom method with the accuracy of machine learning. The result is a one-of-a-kind potential that accurately reproduces the pressure-temperature phase diagram of tin, making the hybrid potential the only interatomic potential that can quantitatively replicate phases transitions in this material under high pressure.
The researchers used a neural-network-based approach to train the machine learning potential using density functional theory calculations. Tin was selected given its complex phase system and its structural, electrical and thermodynamic properties, which have interesting current and potential technological applications in areas such as lithium-ion batteries, solar photovoltaics, hydrogen generation and more.
The researchers noted that this potential demonstrates the necessity of adding an underlying physics model to the machine learning potentials to create a stable interatomic potential. The inclusion of a physics model represents a difference from the general approach, which does not use subject matter expert judgement or underlying materials science to train potentials. Using the new, hybrid approach not only ensures a more accurate potential but also reduces the training data-set size.
The researchers’ method is generally applicable to other elements and multicomponent systems that require a high level of accuracy and can confidently be used to gain insights into deformation mechanisms of complex materials.
Funding and mission
This work was funded by the Advanced Technology Development and Mitigation (ATDM) project within the Advanced Simulation and Computing (ASC) Program.
Reference
“Hybrid interatomic potential for Sn,” Physical Review Materials, 7, 043601 (2023); DOI: 10.1103/PhysRevMaterials.7.043601. Authors: Mashroor S. Nitol, Michael I. Baskes, Khanh Dang, Saryu J. Fensin (Los Alamos National Laboratory); Doyl. E Dickel, Christopher D. Barrett (Mississippi State University).
Technical contact: Saryu Fensin (MPA-CINT)
Materials Science and Technology
Novel deposition technique for reusable vessels in nuclear metallurgical foundries
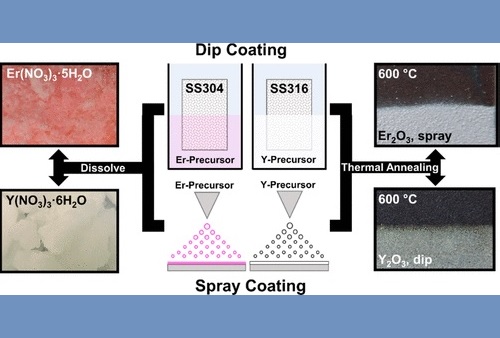
Protective erbia (Er2O3) and yttria (Y2O3) coatings onto stainless steel coupons. Dip coating was shown to yield a higher adhesion strength than spray coating and was further increased if the substrate had preliminary sandblasting.
The casting of molten metals, including f-table elements, is important for nuclear metallurgical foundries. However, the molds and crucibles used suffer from short lifespans due to corrosion, increasing replacement and waste removal costs. The creation of a “reusable” mold — by depositing a protective coating onto the melt-containing surface — is one solution, with rare-earth oxides considered strong candidates due to their properties of non-wettability against molten metals.
In Applied Materials and Interfaces, Los Alamos materials researchers report on a technique that uses chemical solution deposition to apply rare earth-containing precursors to form thick metal oxide coatings onto low-cost substrates. Their technique relies on solution-based procedures used by others in the area of thin film research. The approach is promising, suggesting its role will be significant for application in the foundry.
Specifically, the researchers deposited protective erbia and yttria coatings on stainless steel coupons. Dip and spray coating techniques were tested and compared to a commercial yttria spray. The team observed that solution concentration, solvent choice, micropowder injection, and annealing temperature and ramp profile were critical to the coating’s physical properties. The work, which provides baseline information enabling further refinement in future studies, leveraged the Lab’s expertise in thin films synthesis and characterization, manufacturing science, and foundry operations.
Funding and mission
The work, which supports the Laboratory’s Global Security mission area and its Materials for the Future capability pillar, was funded by Los Alamos’s Laboratory Directed Research and Development program. It was performed in part at the Center for Integrated Nanotechnologies, a DOE Office of Science user facility operated jointly by Sandia and Los Alamos national laboratories.
Reference
“Chemical solution deposition of protective Er2O3 and Y2O3 coatings onto stainless steel for molten metal casting using metal-nitrate precursors,” Applied Materials and Interfaces, 15, 23, 28649 (2023); DOI: 10.1021/acsami.3c05186. Authors: Daniel J. Rodriguez, Alexander S. Edgar, Darrick J. Williams, Ashleigh M. Chov, Douglas R. Vodnik, David J. Ross, Victor P. Siller and Igor O. Usov (Los Alamos National Laboratory).
Technical contact: Daniel Rodriguez, Igor Usov (MST-7)
Physics
Hafnium isomer used to search for dark matter
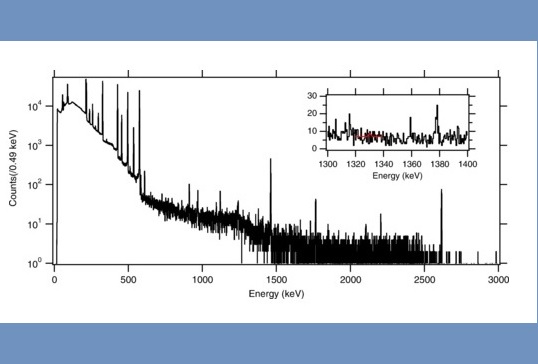
The recorded energy spectrum of gamma-rays emitted from the hafnium-178m source measured with a germanium detector. The structures and peaks in the event distribution are from the know standard decay. The inset shows one of the regions in which a small additional structure would occur if dark matter is abundant enough and has a strong enough coupling. After only 15 minutes of measurement time, the experiment reached its sensitivity. This suggests that with small improvements like background shielding a better result is in reach.
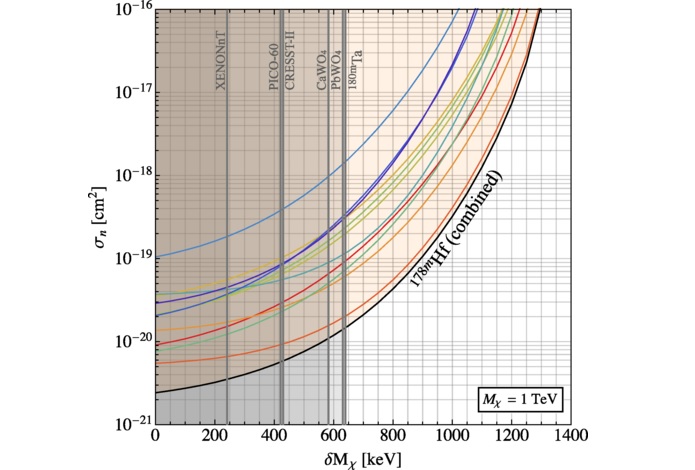
The colored area under the curves is the excluded phase space for this specific type of dark matter. Other larger efforts (gray) like the XENONnT experiment instrument several tons of material and can search for dark matter that interacts much more rarely (smaller cross sections). However, the isomer-based hafnium search allows research to push the boundary towards heavier mass states (colored lines). Each colored curve represents a different dark matter-induced deexcitation path of the isomer. Since none of them showed any hints of dark matter, a combined limit can be calculated.
A research effort led by Los Alamos National Laboratory has used measurements of radiation from a sample of hafnium-178m in an innovative new search for dark matter. As described in Physical Review Letters, the team obtained a sample of the isomer hafnium-178m and employed a germanium detector to search for gamma radiation that would be the result of inelastic scattering with dark matter. The limits obtained for the gamma flux offer constraints on the parameter space of inelastic dark matter and point to possibilities for an expanded science reach with hafnium-178m in future studies.
The research explores the value of using nuclear metastable states as exothermic-reaction targets for dark matter searches. Hafnium-178m, a man-made isotope with a relatively short, 31-year half-life, is a compelling prospect for transference to dark matter searches because it has a large reservoir of available energy for transference to dark matter. The supply of hafnium-178m was fortuitously available at the Laboratory, having been produced at LANL and chemically extracted from radiation samples in the 1980s. The availability of the unique sample made this a special opportunity to work with an isomer that has energy ranges of interest for dark matter studies. Extracted from a tantalum target, the relatively old sample has had other isotopes decay away, leaving a rather pure sample of hafnium-178m.
Hafnium has one of the most energetic state in the isomeric landscape. The isomer’s high metastable state energy allows for competitive measurements with a small-scale, at-surface experiment — an advantage over deep underground, kilo-ton detector experiments often employed in the dark matter field. The reason for this competitiveness lies in the detection mechanisms. Heavy dark matter, up to a thousand times as heavy as a proton, interacts with the hafnium and transfers into a slightly heavier type, while enabling the isomer to decay off the traditional decay path. This new path would reveal itself by emitting gamma-rays, which are not seen under normal circumstances. The team used a germanium detector positioned some four feet away from the sample to investigate if such a measurement is possible. The data from this first experiment and its results were already world-leading in certain parameter spaces.
This effort combined the experimental capabilities at Los Alamos, isotope production chemistry, and dark matter and rare event searches. It combines the work from scientists in the 1980s and opens the door to an array of improvements and options for future measurements that can be investigated. A longer run time and shielded detector, perhaps a high-efficiency gamma-detector array, could improve sensitivity. Deployment of the sample and the detector underground would improve the parameter space conditions since it is expected that the dark matter flux changes deep underground. Further, a larger sample of the hafnium isomer would avail more options for detection, though the costs of production and the logistics of handling the radioactivity of such a sample would have to be weighed against the science reach.
Funding and mission
We gratefully acknowledge support from the U.S. Department of Energy Office of Science and from the Los Alamos National Laboratory’s Directed Research and Development (LDRD) Program for this work. The work supports the Global Security mission area and the Nuclear and Particle Futures capability pillar.
Reference
“Dark Matter Constraints from Isomeric 178mHf,” Physical Review Letters, 131, 141801 (2023); DOI: 10.1103/PhysRevLett.131.141801. Authors: D. S. M. Alves, S. R. Elliott, R. Massarczyk, S. J. Meijer (Los Alamos National Laboratory); H. Ramani (Stanford University).
Technical contact: Ralph Massarczyk (P-1)