Venado's yearlong voyage powers vital AI science
Part three: the supercomputer can help predict materials failure
May 15, 2025
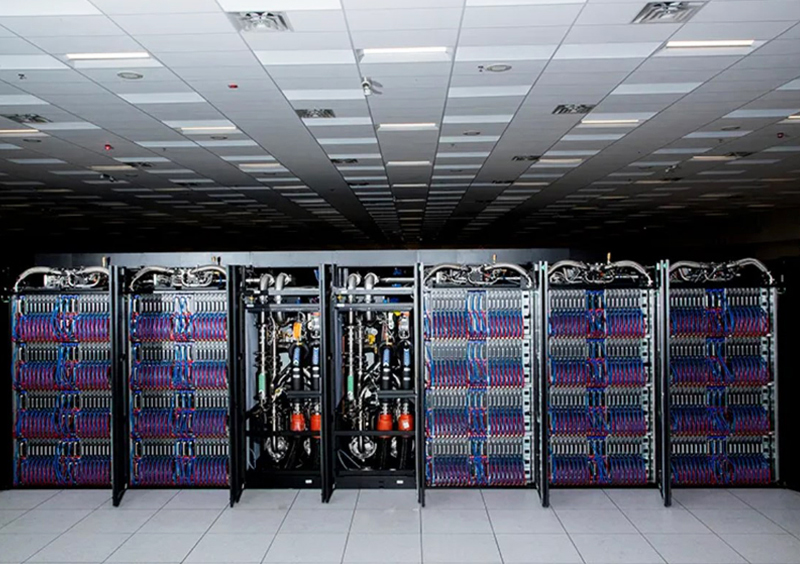
In the year following its April 2024 ribbon-cutting, Venado — the Lab's newest, AI capable supercomputer — racked up an impressive collection of success stories. This four-part series reviews some of the supercomputer's successful applications to high-priority, data-intensive science.
Now, Venado's powerful processing and artificial intelligence capabilities will address the most pressing national security threats as it moves to a classified network.
"The plan for Venado has always been to move it to the secure network," said Mark Chadwick, associate Laboratory director for Simulation, Computation and Theory. "This move happened quickly because of the incredible pace of innovation in AI. These computational and scientific advances for our broad missions will be pursued with our collaborative partners at other national Labs, industry and academia."
Read parts one and two of this series.
Part 3: Billions of AI parameters work to predict materials failure
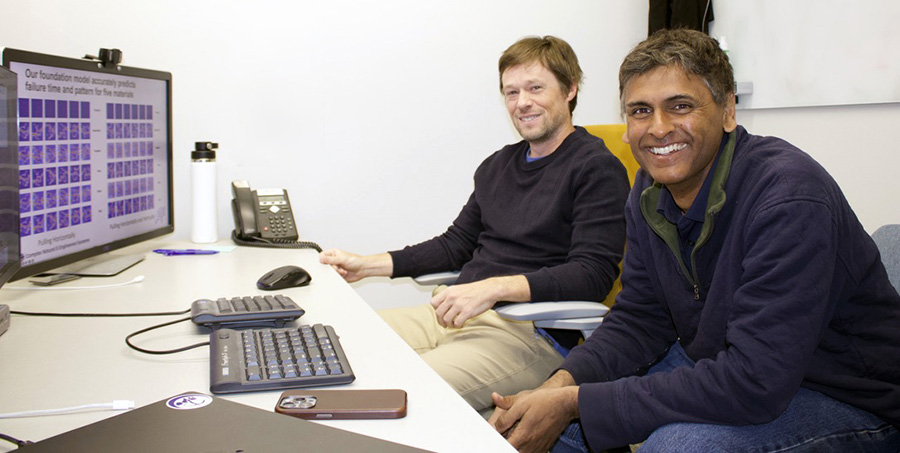
Predicting how and when materials will fail can impact important infrastructure such as bridges, industrial machinery and earthquake-proof buildings. More accurate prediction capabilities will aid scientists and engineers in optimizing material design by helping them consider lighter, stronger, cheaper and more stable options — which can ultimately prevent catastrophic failure. For the Lab, understanding failure points has critical implications for research in energy development, space exploration and weapons design.
"We built a scientific foundation model designed to predict how and when materials will break under stress," said Hari Viswanathan of the Energy and Natural Resources Security group and a co-lead on the project. "This work is crucial for designing stronger structures, improving safety in engineering and understanding fractures in natural materials like rocks or bones."
New approach leads to more materials simulations using fewer resources
To date, most scientific AI models use neural networks with less than a million parameters, which are settings that models can self-adjust, to help them understand and perform tasks better. The utility of these models is greatly reduced since they need to be retrained for each different material, rendering it an impractical exercise. The team's approach shifts the paradigm from the million-parameter models for specific materials to a model that can generalize across multiple materials.
The foundational model is trained on large datasets that simulate material failure under different loading conditions (what kind of forces are applied) and boundary conditions (how materials are supported or constrained). Unlike traditional physics-based simulations, which are computationally expensive, this model can make accurate predictions faster across multiple material types. The system uses multimodal data with different types of inputs and outputs as well as a transformer-based architecture to learn patterns of material fracture across a wide range of conditions, much like the way large language models predict language by "paying attention" to connections of words, ideas and pieces of information.
Venado makes billion-parameter model upgrade possible
Venado's powerful blend of scientific computing and AI capability allowed the team to develop large machine learning models trained on billions of parameters for materials failure research, far outpacing previous research constrained at the million-parameters mark.
Large, billion-parameter neural networks are essential for successful foundation model development. Training these large models has revealed beneficial emergent properties, such as "few-shot learning,” where models can quickly learn new cases that do not require exorbitant retraining.
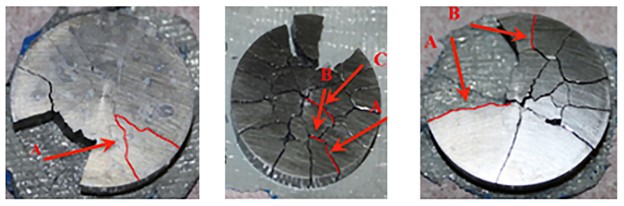
"Our largest model has 3 billion parameters, and we can capture complex fracture behavior that generalizes across five different mission-relevant materials: PBX, tungsten, steel, shale and aluminum," said Dan O'Malley, the project's other co-lead.
An important finding was that, in some cases, even multi-million parameter models showed good generalization. Therefore, Venado was not always deployed to create foundation models. The number of parameters required was highly dependent on the physics being simulated with more parameters needed for ones with higher complexity. Venado's computational power was instrumental in both generating the training data and training the foundation model, while most of the project's physics simulations were then performed on Chicoma, a Lab supercomputer dedicated to open science.
RELATED: Read the AI issue of 1663 Magazine
ChatGPT for science?
With Venado and the team's large-parameter foundation model, the preliminary results on materials have shown significant promise when predicting failure patterns as well as time to failure. The team now has its sights on adapting the foundation model to many different materials and tasks.
"Next, we will determine if we can create a foundation model for broader scientific applications where the data can include experiments and physics-based simulations instead of text and images which have been the industry focus," Viswanathan said. "We also plan on linking our work to the materials discovery work at Los Alamos to design materials that are truly resistant to fracture under extreme conditions."
According to O'Malley, the team can then leverage the different Lab foundation models and AI agents to automate parts of the process.
"Think of it as a ChatGPT for science," he said, describing the hopes of adding a new, powerful tool into the scientific workflow.
LA-UR-25-23993
More from Los Alamos National Laboratory:
Los Alamos partners with OpenAI to advance national security
Can a single AI model advance any field of science?
Contact
Public Affairs | media_relations@lanl.gov