Venado's yearlong voyage powers vital AI science
The supercomputer has made a profound impact
May 1, 2025
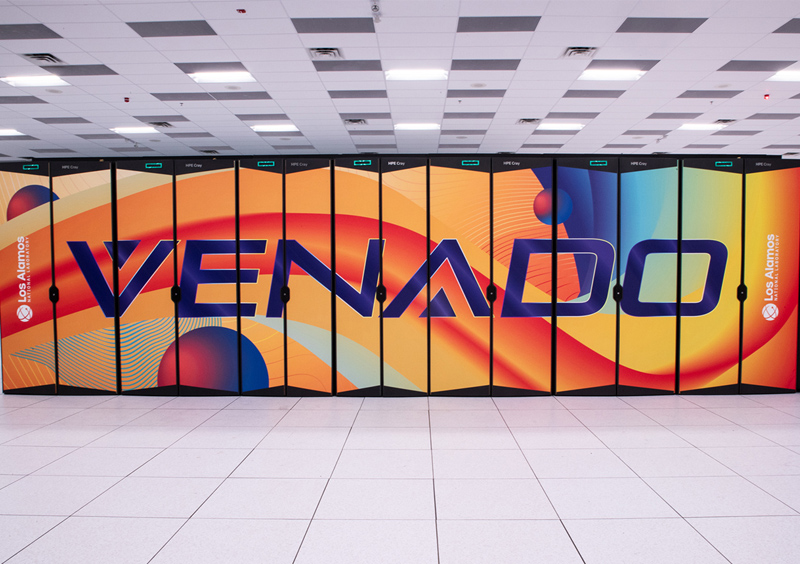
In the year following its April 2024 ribbon cutting, Venado — the Lab's newest, AI capable supercomputer — racked up an impressive collection of success stories. This four-part series reviews some of the supercomputer's successful applications to high-priority, data-intensive science.
Now, Venado's powerful processing and artificial intelligence capabilities will address the most pressing national security threats as it moves to a classified network.
"The plan for Venado has always been to move it to the secure network," said Mark Chadwick, associate Laboratory director for Simulation, Computation and Theory. "This move happened quickly because of the incredible pace of innovation in AI. These computational and scientific advances for our broad missions will be pursued with our collaborative partners at other national Labs, industry and academia."
Part 1: Rapid development and deployment of complex models empowers robust material simulations
For Theoretical division scientists, machine-learning (ML) algorithms are helping "peek behind the curtain" to understand the nature of materials down to the level of individual atoms — a process that is essential when developing new materials with improved capabilities. This work seeks to develop accurate ML models to predict the behavior of materials in areas critical to the Lab’s mission. Predicting the behavior of materials used for fusion reactor walls — essential before fusion power can become a commercial reality — is a prime example.
Energy is critical when describing materials. Atoms in materials are constantly pushing and pulling against each other, and the amount of energy generated by this motion can reveal how stable the material is, which configuration is likely to occur, how atoms will move over time and what materials might form under certain conditions. Physicists and materials scientists use mathematical functions called "interatomic potentials" to describe how atoms interact with each other.
These traditional models can be simple and easy to interpret but are not always highly accurate — especially for complex materials. This is where Machine-Learned Interatomic Potentials (MLIAPs) come into play. This type of model utilizes training datasets to learn the energetics of materials using quantum mechanical calculations to create relationships between atomic configurations and their energies.
"The unique combination of the Lab's expertise on materials in extreme conditions and our ability to develop new tools for high-performance computing, like Venado, dramatically lowers the bar for generating new models that meet our specific needs," said Danny Perez, of the Theoretical division at Los Alamos. "This puts us in an enviable position where we can quickly chart unexplored materials in search of solutions to mission needs."
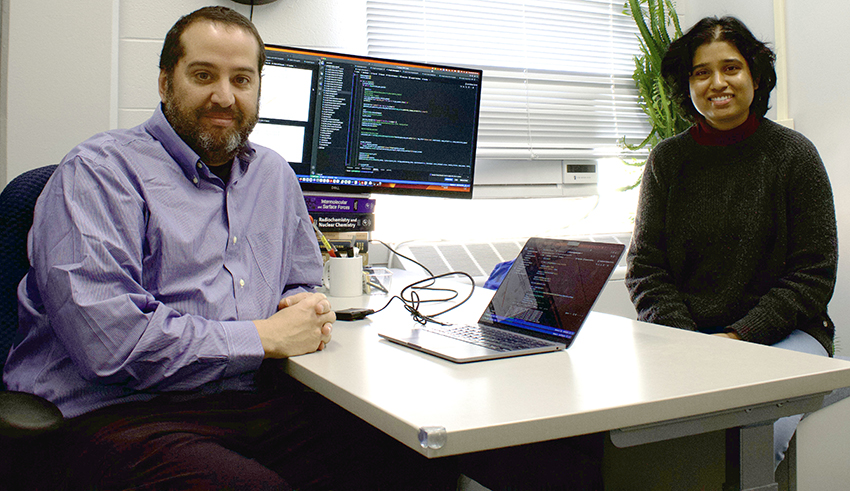
Novel approach addresses challenges in creating accurate MLIAPs
A key challenge for researchers: Because they require lots of quantum training data, developing MLIAP models can be extremely time consuming and costly. The process calls for extensive testing and validation. Multiple rounds of iterative improvements are often required because the models can become erratic when asked to predict the energetics of atomic configurations that stray away from the training dataset. This slows down the process, dramatically limiting the number of materials that can be considered and simulated.
Perez noted that for the past 20 years, researchers had to choose between running an accurate simulation for a very short time on a very small system or running a large simulation at lower levels of accuracy.
The team addressed this by developing new methods to maximize the amount and diversity of information in training datasets without requiring human intervention. The novel approach fills "holes" in the dataset where the MLIAP would otherwise have insufficient information to make accurate predictions. This creates uniquely robust models that maintain their accuracy even in extreme conditions where unusual atomic arrangements — difficult to predict even for experts — are encountered. Focusing only on information-rich configurations minimizes the amount of quantum calculations required to train good MLIAPs.
The team also trained and tested a series of autonomous computational workflows for managing substantial amounts of quantum calculations. This provided researchers with quick access to a menu of various models with different accuracy-speed tradeoffs, which could then be applied to materials being studied. This all came together to significantly reduce the time needed to develop new MLIAPs.
"Using MLIAPs, we are able to have our cake and eat it, as affordable ML models are catching up with direct quantum calculations," Perez said. "This is extremely exciting and hints at a dramatically different way of doing research where simulations aren't constrained by the models that are available. Instead, we can be truly creative with the materials we consider."
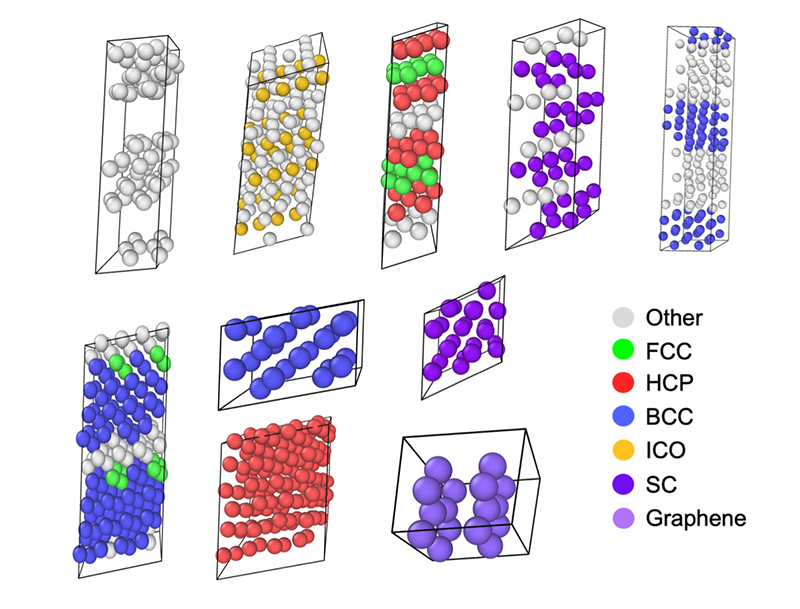
Venado facilitates MLIAP development at super speed
By using Venado to rapidly generate data and train models, Perez and his team took the human completely out of the loop while creating new high-quality MLIAPs in a fraction of the time. With Venado's powerful GPUs, the researchers carried out tens of thousands of quantum calculations a day, enabling the creation of new ML models within 24 hours. Without Venado, the calculations would have taken weeks for each different model, making such a study prohibitively slow.
The unparalleled power of the GPUs and MLIAPs allowed the team to create "digital twins" — one-to-one models at the atomic scale used to simulate materials — that can predict the energy and forces of atoms based only on their positions. The resulting responsiveness was unprecedented, enabling the team to explore a significant number of different materials.
Training a single model
Ultimately, Perez and his team — in collaboration with Interdisciplinary Centre for Advanced Materials Simulation in Germany — aim to train a single model that can describe any material at once, even in extreme conditions, eliminating the need to use separate models for different materials. Much of this work was made possible with the use of Venado's unique characteristics.
LA-UR-25-23993
Contact
Public Affairs | media_relations@lanl.gov