The Advanced Simulation and Computing (ASC) Program supports the Department of Energy's National Nuclear Security Administration (NNSA) Defense Programs by developing simulation capabilities and deploying computing platforms to analyze and predict the performance, safety, and reliability of nuclear weapons and to certify their functionality in the absence of nuclear testing. The ability to model the extraordinary complexity of nuclear weapons systems is an essential element of the Stockpile Stewardship Program (SSP).
Nuclear weapon simulation and computing
Ensuring the safety and reliability of the nation's nuclear weapons stockpile
The ASC Program at Los Alamos delivers leading-edge computer systems, sophisticated physics and engineering codes, physical data, and uniquely qualified staff to support the science-based SSP. ASC tools enable nuclear weapons scientists and engineers to gain a comprehensive understanding of the weapons lifecycle—design, development, production, certification, life extension, retirement, and dismantlement—and take on the most challenging simulation problems of today and tomorrow.
Los Alamos works in partnership with Lawrence Livermore National Laboratory and Sandia National Laboratories to share long-distance computing capabilities so that SSP staff have access to the largest systems at any of the labs. ASC also collaborates with universities to advance the state-of-the-art in computational physics, including providing university students with access to ASC supercomputers.
Program elements
Integrated Codes
Integrated Codes (IC) contain the mathematical descriptions of the physical processes relating to nuclear weapon systems and describe what the nation knows about how nuclear weapons function.
Physics and Engineering Models
The Physics and Engineering Models (PEM) subprogram provides the models and databases used in simulations supporting the U.S. stockpile.
Verification and Validation
The Verification and Validation (V&V) subprogram provides evidence that the models in the codes produce mathematically credible answers that reflect physical reality.
Computational Systems and Software Environments
The Computational Systems and Software Environments (CSSE) subprogram builds integrated, balanced, and scalable computational capabilities to meet the predictive simulation requirements of the NNSA.
Facility Operations and User Support
The Facility Operations and User Support (FOUS) subprogram provides two critical enablers for the effective use of ASC tri-lab computing resources: 1) physical facility and operational support for reliable, cross-lab production computing and storage environments, and 2) a suite of user services.
Related Videos
All VideosComputing News
All News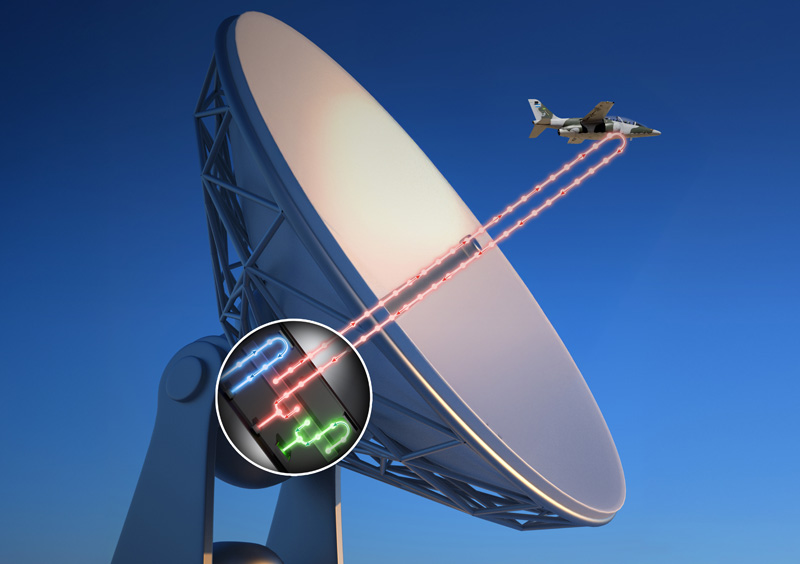
Quantum remote sensing approach overcomes quantum memory problem
Path identity the key to scheme that can unlock national security and communications applications
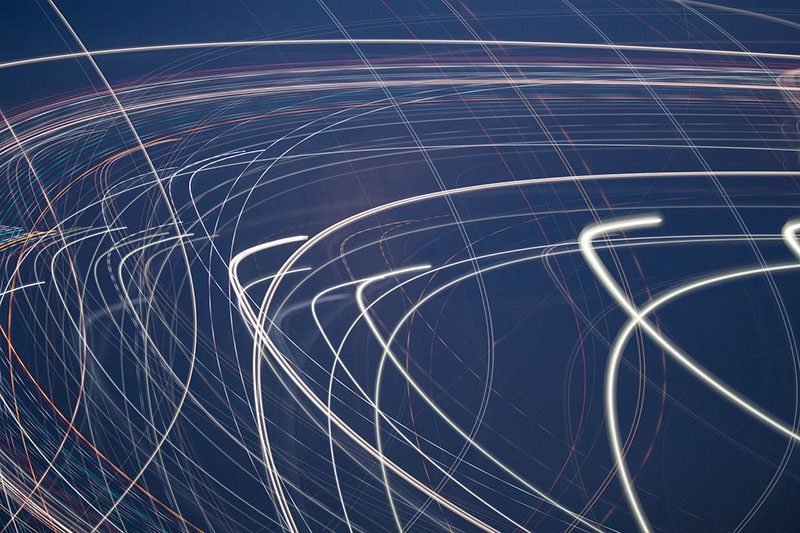
University of Michigan, Lab to jointly develop Michigan-based AI research center
This U-M press release highlights how a partnership expands research collaboration
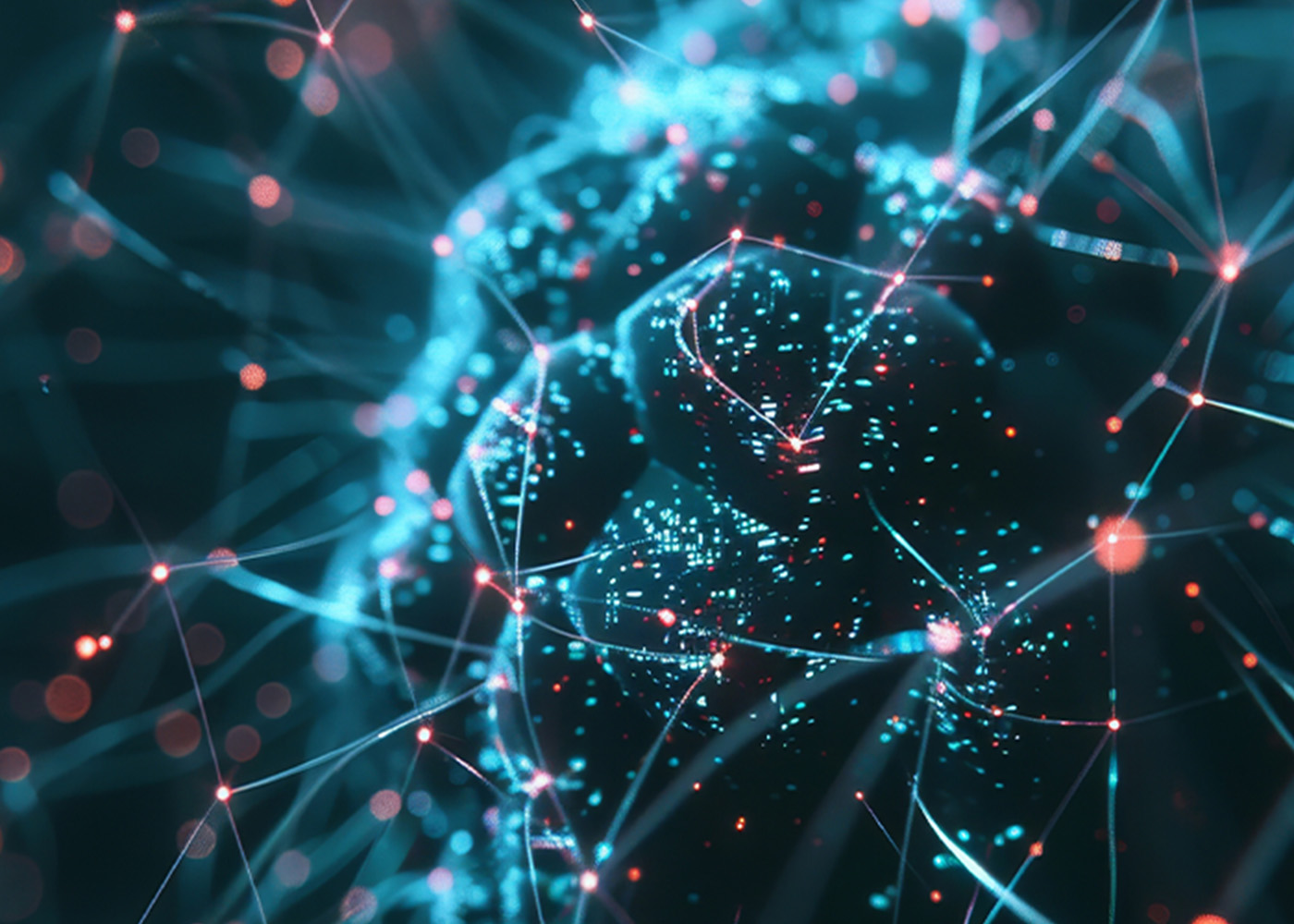
AI algorithms deployed on-chip reduce power consumption of deep learning applications
Brain-inspired algorithm on neuromorphic hardware reduces cost of learning