Los Alamos team cracks the code on the bane of quantum machine learning algorithms
Barren plateaus have long plagued progress in the field of variational quantum computing, but their understanding has been limited — until now
August 26, 2024
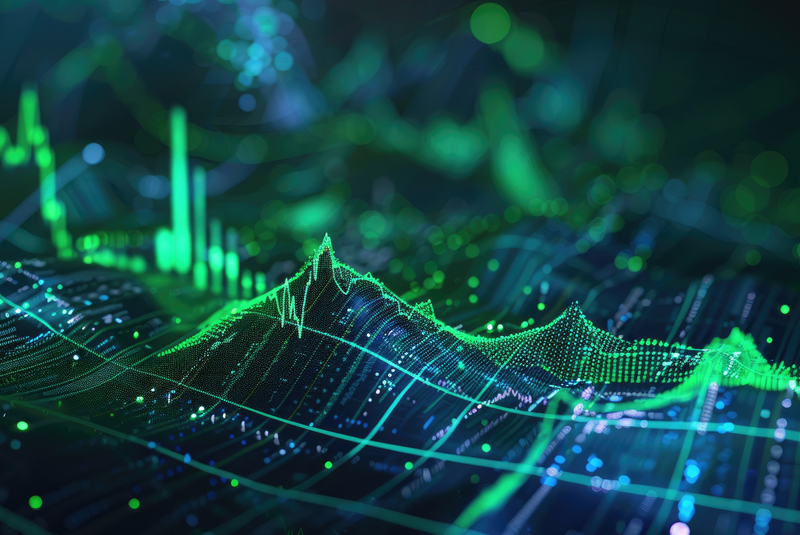
Quantum computers are still a nascent technology, but researchers are busy building complex machine learning algorithms to test the capabilities of quantum learning. Sometimes, however, their algorithms hit a mysterious dead end; a mathematical path from which there is no way forward or backward — the dreaded barren plateau.
“Understanding the problem of barren plateaus was regarded as the key to unlocking quantum machine learning, and our team has worked on this for five years,” said Marco Cerezo, the Los Alamos team’s lead scientist. “The major issue was that we knew these barren plateaus existed, but we didn't really know what unified all the sources of this phenomenon. But what we have done now is characterize, mathematically, why and when barren plateaus occur in variational quantum algorithms.”
Rather than ones and zeros, as conventional computers use, quantum computers use qubits, which take advantage of quantum phenomena such as superposition — an ability to exist in several states at the same time. It is believed that quantum computers, when paired with quantum algorithms, will help humanity solve certain types of problems that were previously too difficult, or took too long, for conventional computers.
Barren plateaus were a little-understood but common problem in quantum algorithm development. Sometimes, after months of work, researchers would run their algorithm and it would unexpectedly fail. Scientists had developed theories as to why barren plateaus exist and had even adopted sets of practices to avoid them. But no one knew the underlying cause of this mathematical equivalent of a dead end.
With a precise characterization of barren plateaus, scientists now have a set of guidelines to follow when creating new quantum algorithms. This will prove essential as quantum computers scale in power, from a maximum of 65 qubits three years ago to computers with more than 1,000 qubits in development today.
This breakthrough — a unified theory of barren plateaus — removes the guesswork of building quantum machine learning algorithms that can lead to wasted time and resources. It also removes one of the largest challenges facing the field of quantum computing.
Proving a theory
Barren plateaus most often occur in optimization algorithms. A basic optimization problem is that of a traveling salesperson who, after being given a list of addresses, must find the most efficient route to each house. To solve such optimization problems, a quantum computer encodes the task of finding the optimal path into a learning problem, where one must navigate an optimization landscape composed of peaks and valleys. The peaks represent bad solutions, whereas the valleys are associated with good results.
When a barren plateau is present, the peaks and valleys are separated by wide regions of flat, featureless plains. The optimization algorithm can get stuck in these plateaus without any way of knowing which way to navigate. Scientists had studied this phenomenon, but only in specific algorithm architectures. And because no unified theory existed to explain or predict barren plateaus, researchers were left to guess.
“We had conjectured in a previous paper that there are clear connections between certain algebraic properties in these optimization algorithms and the presence of barren plateaus,” said Martin Larocca, who came to Los Alamos as a postdoc specifically to work on the barren plateau problem. “Our latest paper proves this conjecture, and developed a kind of recipe to follow that allows researchers to test their algorithm for the presence of barren plateaus.”
The team accomplished this by developing an equation that was able to predict barren plateaus in any quantum optimization algorithm. Even more, their equation uncovered new sources of barren plateaus.
They discovered generic quantum optimization algorithms that set out to solve a variety of tasks will more frequently encounter barren plateaus. Essentially, specialization, rather than generalization, is the key to avoiding barren plateaus. This breakthrough allows scientists to understand and unify all known sources of barren plateaus, and thus avoid them as they build their algorithms.
This research represents the first time anyone has successfully developed a unified, mathematical approach to identifying barren plateaus. The results will have a far-reaching impact in the field of quantum computing, which has rapidly developed in recent years.
The research has been published in the journal Nature Computational Science. This work was conducted together with researchers at University of California, Davis; North Carolina State University; and Pacific Northwest National Laboratory.
Paper: “A Lie Algebraic Theory of Barren Plateaus for Deep Parameterized Quantum Circuits.” Nature Communications. DOI: 10.1038/s41467-024-49909-3
Funding: Laboratory Directed Research and Development Program
LA-UR-24-28066
Contact
Weston Phippen | jwphippen@lanl.gov